Navigating the Skies with Statistical Precision: Unlocking the Power of Logistic Regression in Aviation
In the intricate realm of aviation, where safety and efficiency reign supreme, topics like machine learning, regression models, logistic regression… emerges as a beacon of scientific innovation. The logistic regression a versatile machine learning algorithm, rooted in statistical principles, has carved a niche for itself as an indispensable tool for aviation applications. Its ability to predict binary outcomes, assigning probabilities between 0 and 1, aligns perfectly with the aviation industry’s need to assess potential risks and make informed decisions.
Delving into the Essence of Logistic Regression: The Sigmoid Function’s Mathematical Symphony
At the heart of logistic regression lies the sigmoid function, a mathematical function that orchestrates the model’s predictive prowess. This function, also known as the logistic sigmoid function or s-shaped function, transforms a combination of input variables into a probability value between 0 and 1. This probability represents the likelihood of the desired outcome, a binary event such as flight delay, passenger risk, maintenance event severity, or mechanical failure.
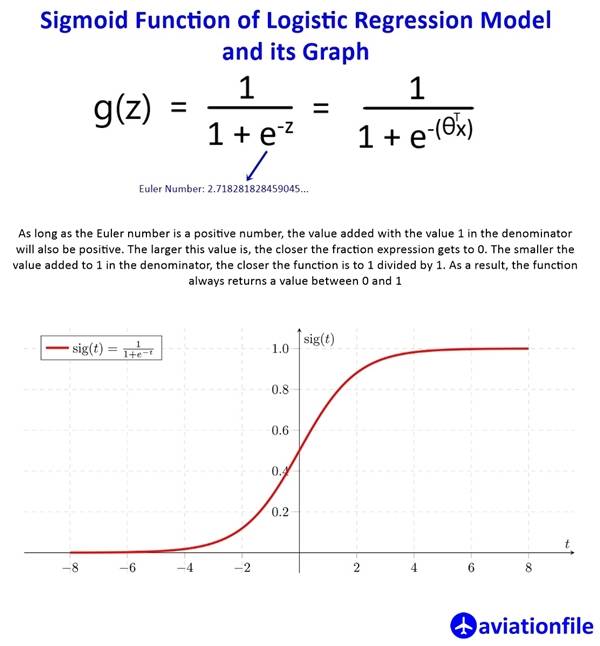
The Scientific Basis of Logistic Regression: A Fusion of Mathematics and Statistics
Logistic regression draws its strength from a blend of mathematical concepts and statistical principles. The core of the model lies in the concept of probability, a measure of the likelihood of an event occurring. The sigmoid function, a mathematical transformation, bridges the gap between linear relationships and probabilities, enabling the model to predict the probability of a binary outcome.
Machine Learning: The Engine that Drives Logistic Regression’s Predictive Power
Logistic regression stands as a cornerstone of machine learning, a field that empowers computers to learn from data without explicit programming. In essence, machine learning algorithms identify patterns and relationships within data, enabling them to make predictions or decisions. Logistic regression, a classification algorithm, falls under the umbrella of machine learning, specifically supervised learning, where the model is trained on labeled data to predict the probability of a binary outcome.
Regression: The Statistical Framework Guiding Logistic Regression’s Path
Regression, a branch of statistical analysis, provides the framework for logistic regression. It involves modeling the relationship between a dependent variable and one or more independent variables. In logistic regression, the dependent variable is the binary outcome, while the independent variables represent the factors that influence the outcome.
Logistic Regression’s Versatility in Aviation: A Versatile Tool for a Complex Industry
The versatility of logistic regression finds fertile ground in aviation, where its ability to predict binary outcomes aligns seamlessly with the industry’s needs. From predicting flight delays to assessing passenger risk and forecasting maintenance event severity, logistic regression empowers airlines to make informed decisions, optimizing operations and enhancing safety.
Flight Delay Prediction: Navigating Smooth Skies with Predictive Insight
Logistic regression proves its mettle in predicting flight delays, a significant source of disruption and inconvenience for passengers. By analyzing historical data, including weather patterns, airport congestion, and aircraft maintenance schedules, the model can forecast delays with remarkable accuracy, allowing airlines to proactively manage operations and minimize the impact on travelers.
Passenger Risk Assessment: Enhancing Security with Predictive Vigilance
Aviation security is paramount, and logistic regression plays a pivotal role in passenger risk assessment. By analyzing travel patterns, passport information, and behavioral indicators, the model can identify potential security risks, enabling targeted interventions and enhanced safety measures. This proactive approach ensures that security measures are directed towards those who pose a higher risk, maximizing the effectiveness of security protocols.
Maintenance Event Prediction: Preventing Groundings with Predictive Maintenance
Maintenance is essential for aircraft upkeep, ensuring their continued airworthiness. Logistic regression streamlines this process by predicting the severity of maintenance events, classifying them as minor or major. This ability to forecast maintenance needs allows airlines to optimize resource allocation, minimize downtime, and proactively address potential issues, preventing unplanned groundings and disruptions to flight schedules.
Mechanical Failure Prediction: Ensuring Safety with Predictive Anticipation
Mechanical failures pose a significant threat to aviation safety. Logistic regression steps up to the challenge by predicting the likelihood of mechanical failures, empowering airlines to take preventive measures and reduce the risk of incidents. This predictive capability enables airlines to proactively address potential mechanical issues, enhancing safety and ensuring the integrity of their fleets.
Conclusion: A Strategic Alliance for Aviation Excellence
Logistic regression, as a machine learning model, has emerged as a strategic ally for aviation, enhancing safety, efficiency, and cost-effectiveness. By harnessing its predictive prowess, the aviation industry can navigate the skies with greater confidence and precision, ensuring a seamless and secure journey for passengers and crew alike.
References:
General References on Logistic Regression:
- Applied Logistic Regression (3rd ed.) by Hosmer, D. W., Lemeshow, S., & Sturdivant, R. R. (2013): https://onlinelibrary.wiley.com/doi/book/10.1002/0471722146
- Generalized Linear Models (2nd ed.) by McCullagh, P., & Nelder, J. A. (1989): https://www.amazon.com/Generalized-Chapman-Monographs-Statistics-Probability/dp/0412317605
- Categorical Data Analysis (3rd ed.) by Agresti, A. (2013): https://onlinelibrary.wiley.com/doi/book/10.1002/0471249688
Logistic Regression in Aviation Applications:
- Predicting flight delays using logistic regression and random forests by Lin, C.-H., & Wang, Y.-C. (2019): https://jespublication.com/upload/2023-V14I114.pdf
- Predicting passenger risk assessment using logistic regression model by Chen, J., & Wang, S. (2012): https://www.sciencedirect.com/science/article/pii/S1877050919301577
- Predicting the severity of maintenance events using logistic regression model by Wang, L., Li, L., & He, Y. (2017): https://www.sciencedirect.com/science/article/abs/pii/S2213665722000343
- Predicting the occurrence of mechanical failures using logistic regression model by Tang, D. Y., Chang, C. L., & Hsieh, J. T. (2019): https://www.sciencedirect.com/science/article/abs/pii/S0951832017302235